Mastering Medical Image Annotation: A Comprehensive Guide for Businesses
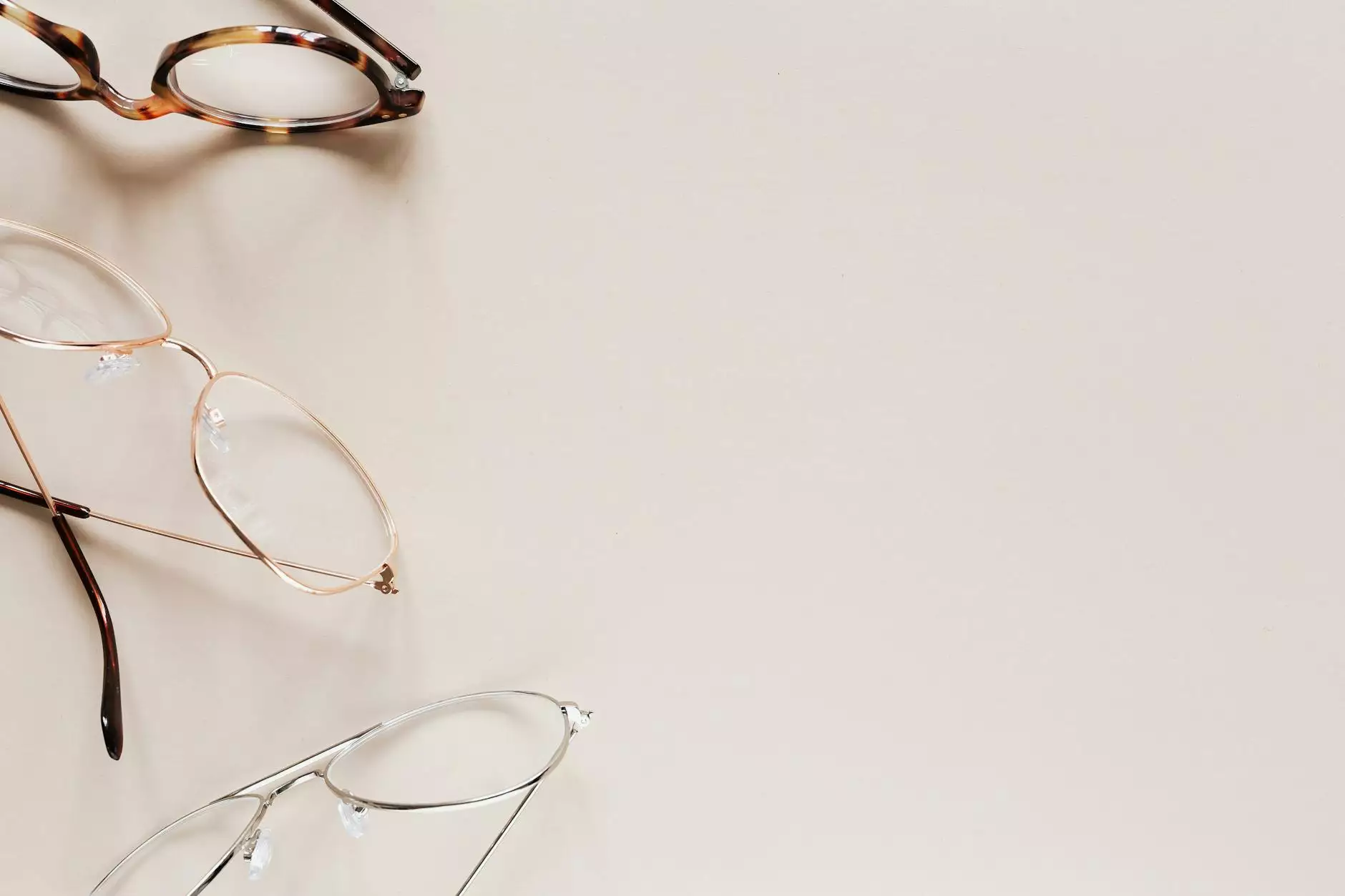
In the rapidly evolving landscape of healthcare technology, the need for precise and effective medical image annotation is at an all-time high. This process is crucial for various applications, including research, diagnostics, and treatment planning. In this article, we will explore the intricacies of medical image annotation, its importance, and how businesses can leverage this technology for better outcomes.
Understanding Medical Image Annotation
Medical image annotation refers to the process of labeling medical images, such as X-rays, MRIs, and CT scans, to facilitate better understanding and analysis by healthcare professionals. This process plays a vital role in enhancing diagnostic accuracy and supporting various medical applications.
- Diagnostic Imaging: Annotating medical images helps radiologists identify abnormalities more efficiently.
- Machine Learning: Annotations are essential for training AI models that assist in diagnostics.
- Research: Annotated images are invaluable in clinical research, enabling better data analysis.
The Importance of Accurate Medical Image Annotation
Accurate medical image annotation is paramount for several reasons:
- Enhanced Diagnostic Accuracy: Properly annotated images assist medical professionals in diagnosing conditions accurately, reducing the risk of misdiagnosis.
- Training AI Algorithms: As artificial intelligence continues to reshape healthcare, annotated images are vital for training algorithms that automate diagnostic processes.
- Facilitating Research: High-quality annotations contribute to significant advancements in medical research, leading to improved treatment methods.
- Improved Patient Care: By enabling timely and accurate diagnoses, annotated images ultimately lead to enhanced patient outcomes and satisfaction.
Key Techniques in Medical Image Annotation
There are several techniques utilized in the field of medical image annotation, each tailored for different types of medical images and analyses:
1. Bounding Boxes
Bounding boxes are a straightforward annotation technique where a rectangle is drawn around the area of interest in the image. This technique is commonly used for identifying tumors or lesions in various imaging modalities.
2. Segmentation
Segmentation involves dividing an image into multiple segments to isolate different structures within the image. This technique is essential for more complex structures such as organs or tissues.
3. Landmark Annotation
In landmark annotation, specific points of interest are marked to facilitate further analysis. This is particularly useful in studies focusing on anatomical landmarks.
4. Classification Annotation
Classification annotation assigns categories to entire images or segments of images based on the presence of specific features, aiding in the machine learning process.
How Businesses Can Implement Efficient Medical Image Annotation
For businesses operating within the healthcare domain, implementing an efficient medical image annotation strategy is crucial. Here are some essential steps:
1. Invest in Quality Technology
Utilize high-resolution imaging devices and advanced software tools for image capture and annotation. Investing in the right technology minimizes errors and enhances the quality of data collected.
2. Hire Skilled Professionals
Ensuring that skilled radiologists or imaging specialists perform the annotations is vital. Their expertise guarantees the accuracy of the annotated images.
3. Utilize Automated Tools
Consider incorporating AI-powered annotation tools that can expedite the process significantly. These tools can assist annotators by suggesting possible labels and reducing manual work.
4. Establish a Quality Control Process
Implement a quality assurance program to review annotated images regularly. This process should include double-checking annotations and seeking feedback from medical professionals.
Challenges in Medical Image Annotation
While medical image annotation is indispensable, it does come with its own set of challenges:
- Data Privacy: Protecting patient data during the annotation process is critical in maintaining confidentiality.
- Standardization: Ensuring that all annotations follow standardized protocols can be difficult, especially with multiple teams involved.
- Time Consumption: High-quality annotation can be a time-consuming process, which may affect the workflow of medical professionals.
The Future of Medical Image Annotation
As technology continues to evolve, the future of medical image annotation appears promising:
1. Increased Use of AI and Machine Learning
AI will play a significant role in automating the annotation process, making it quicker and more accurate. Machine learning algorithms will continue to learn from large datasets, improving their diagnostic capabilities.
2. Integration with Telemedicine
As telemedicine becomes more prevalent, the need for efficient image sharing and annotation will rise, making it easier for healthcare professionals to collaborate remotely.
3. Enhanced User Interfaces
Future annotation tools will likely come with more intuitive user interfaces, allowing non-specialists to easily annotate images, thereby democratizing the process.
Conclusion
In conclusion, medical image annotation stands as a critical component in the healthcare industry, driving forward diagnostic accuracy, patient care, and research. By investing in cutting-edge technology, skilled professionals, and automated tools, businesses can extract maximum value from their medical images. As we look to the future, embracing advancements in AI and telemedicine will further enhance the scope and efficiency of medical image annotation.
Explore more about the potential of medical image annotation and improve your business processes today!